AI plays a crucial role in propensity score estimation as it can discern underlying patterns between treatments and confounding variables through machine learning, without explicit programming.
This insight has gone through numerous updates as the information and use of AI-driven propensity scores evolved. In many cases, writers give a brief overview of the what of a tool. Today, we are going way beyond “what the sausage tastes like” to “how the sausage is made” Tectonic hopes you will enjoy learning how propensity models and AI driven propensity scores improve your data.
Propensity Model in Artificial Intelligence: Propensity modeling generates a propensity score, representing the probability that a visitor, lead, or customer will take a specific action. For instance, a propensity model, using data science or machine learning, can help predict the likelihood of a lead converting to a customer. AI-driven propensity scores take an existing data model and improve its predictions, speed, and analysis with AI.
Propensity Score in CRM: In CRM, a propensity score is the model’s probabilistic estimate of a customer performing a specific action. Grouping customers by score ranges allows for effective comparison and analysis within each bucket.
Enhancing Propensity Modeling with AI: Traditional statistical propensity models might lack accuracy, but integrating machine learning technologies, as demonstrated by Alphonso, can significantly optimize ad spend and increase prediction accuracy from 8% to 80%. That’s a whopping 72% improvement.
Propensity Modeling Overview: Propensity modeling involves predictive models analyzing past behaviors to forecast the future actions of a target audience. It identifies the likelihood of specific actions, aiding in personalized marketing.
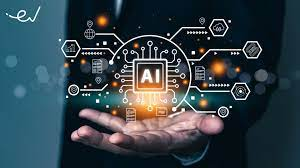
Role of Machine Learning in Propensity Models: Propensity models rely on machine learning algorithms, acting as binary classifiers predicting whether a certain event or behavior will occur. Logistic regression and Classification and Regression Tree Analysis are common methods for calculating propensity scores.
Characteristics of Effective Propensity Models: For robust predictions, propensity models should be dynamic, scalable, and adaptive. Dynamic models adapt to trends, scalable for diverse predictions, and adaptive with regular data updates.
Propensity Modeling Applications: Propensity models find applications in predicting customer behavior, such as purchasing, converting, churning, or engaging. Real-time predictions, data analysis, and AI integration contribute to successful implementations. AI-driven propensity scores are extremely useful in that they can be coupled with many other models to give additional insights to your data.
Types of Propensity Score Models: Various models include propensity to purchase/convert, customer lifetime value (CLV), propensity to churn, and propensity to engage. Combining models can enhance the effectiveness of marketing campaigns.
When to Use Propensity Scores: Propensity scores are beneficial when random assignment of treatments is impractical. They help estimate treatment effects in observational studies, providing an alternative to traditional model-building methods.
Limitations of Propensity Score Methods: While propensity scores help achieve exchangeability between exposed and unexposed groups, they do not claim to eliminate confounding due to unmeasured covariates. Findings from observational studies must be interpreted cautiously due to potential residual confounding.
Content updated October 2021.
Content updated February 2024.