Traditional lead scoring relies on predefined criteria and subjective assumptions, whereas predictive lead scoring (PLS) harnesses machine learning algorithms to analyze extensive data and identify key predictors of lead quality. Traditional lead scoring only learns from data if you revise your scoring methodology for it. Predictive lead scoring constantly reworks the machine learning model based on more and newer data. Traditional lead scoring can be impacted by human error and bias. PLS analyzes from historical data eliminating bias and error.
PLS employs a machine learning model to assign scores to open leads based on historical data, enabling sales teams to prioritize effectively and improve lead qualification rates while reducing the time spent on lead qualification.
Discover how AI can elevate PLS to new heights and transform various organizational functions amidst shrinking budgets and heightened performance expectations across sales and marketing teams.
Key Benefits of Predictive Lead Scoring:
- Enhanced Collaboration Between Marketing and Sales: By aligning on lead quality rather than quantity, predictive lead scoring fosters teamwork between marketing and sales departments, driving improved outcomes.
- Time Savings: Automated lead scoring eliminates manual vetting, allowing teams to focus on generating and nurturing quality leads.
- Elimination of Human Bias: Algorithms remove subjective biases from lead qualification, ensuring objective decision-making.
- Real-Time Results: Once set up, predictive lead scoring delivers rapid, data-driven lead insights compared to human-driven processes.
- Continuous Improvement: As more data is gathered, PLS models evolve and become more accurate over time.
PLS leverages data science and machine learning to analyze and predict future outcomes based on historical and current data, guiding businesses in identifying high-potential leads and optimizing resource allocation.
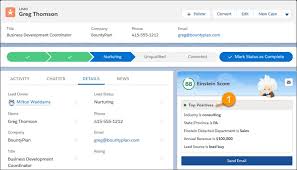
Implementing Predictive Lead Scoring:
- Consider which lead scoring modes (manual, predictive, AI-assisted) are supported.
- Automate data cleaning and preparation for improved model accuracy.
- Ensure transparency in the lead scoring process, enabling insights into scoring factors and outcomes.
AI CRM and PLS:
AI-enabled CRM platforms like Salesforce’s Einstein Lead Scoring automate lead scoring processes, leveraging extensive data to predict lead quality and prioritize effectively for sales and marketing teams.
Benefits of Predictive Lead Scoring:
- Enhanced Collaboration: PLS aligns marketing and sales efforts based on lead quality.
- Time Efficiency: Automation saves time spent on lead vetting, enabling teams to focus on high-potential leads.
- Objective Decisions: Removes human bias from lead qualification, ensuring accuracy and fairness.
- Rapid Insights: Delivers real-time data-driven insights for quick decision-making.
- Continuous Improvement: Evolves and improves over time with more data and refined algorithms.
AI and Machine Learning in Lead Scoring:
AI and machine learning enhance lead scoring by analyzing vast data sets, identifying patterns, and predicting behaviors for more accurate lead qualification and prioritization. A data-driven enterprise is a smarter enterprise acting on data and insights.
Salesforce’s Intelligent Lead Scoring:
Salesforce’s Einstein Lead Scoring automates lead scoring processes within Sales Cloud and Marketing Cloud, providing tailored metrics and insights for informed decision-making.
Generative AI and Predictive Lead Scoring:
Generative AI streamlines processes like email personalization and content creation, enhancing marketing effectiveness and productivity.
Good PLS with AI and machine learning transforms lead management by leveraging data insights for efficient and accurate lead qualification, ultimately driving improved sales and marketing performance.
🔔🔔 Follow us on LinkedIn 🔔🔔