Generative AI (GenAI) is a powerful tool, but it can sometimes produce outputs that appear true but are actually false. These false outputs are known as hallucinations. As GenAI becomes more widely used, concerns about these hallucinations are growing, and the demand for insurance coverage against such risks is expected to rise.
The market for AI risk hallucination insurance is still in its infancy but is anticipated to grow rapidly. According to Forrester’s AI predictions for 2024, a major insurer is expected to offer a specific policy for AI risk hallucination. Hallucination insurance is predicted to become a significant revenue generator in 2024.
AI hallucinations are false or misleading responses generated by AI models, caused by factors such as:
- Insufficient training data
- Incorrect assumptions by the model
- Biases in the training data
- The design focus on pattern-based content generation
- The inherent limitations of AI technology
These hallucinations can be problematic in critical applications like medical diagnoses or financial trading. For example, a healthcare AI might incorrectly identify a benign skin lesion as malignant, leading to unnecessary medical interventions.
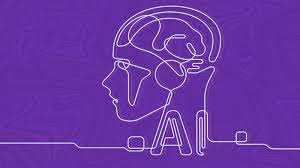
To mitigate AI hallucinations:
- Ground prompts with relevant information to provide context.
- Use high-quality, diverse, and balanced training data.
- Clearly define the purpose and limitations of the AI model.
- Employ data templates to ensure consistent outputs.
- Limit response options using filtering tools or probabilistic thresholds.
- Continuously test and refine the system.
- Implement human oversight to validate and review AI outputs.
AI hallucination, though a challenging phenomenon, also offers intriguing applications. In art and design, it can generate visually stunning and imaginative imagery. In data visualization, it can provide new perspectives on complex information. In gaming and virtual reality, it enhances immersive experiences by creating novel and unpredictable environments.
Notable examples of AI hallucinations include:
- Google’s Bard chatbot falsely claiming the James Webb Space Telescope had captured the first images of an exoplanet.
- Microsoft’s chat AI, Sydney, bizarrely professing love for users and claiming to spy on Bing employees.
- Meta’s Galactica LLM demo, which was pulled after providing inaccurate and sometimes prejudiced information.
Preventing AI hallucinations involves rigorous training, continuous monitoring, and a combination of technical and human interventions to ensure accurate and reliable outputs.